Application of Big Data in construction
26 February 2024Example of Big Data based on CAD (BIM) data
26 February 2024Publicly available data on over one million building permits (records in two datasets) from the “San Francisco Department of Buildings” allows us to use this table-dataset to analyze not only construction activity in the city, but also to critically examine recent trends and history of the construction industry SF over the past 40 years, from 1980 to 2019.
The code examples that were used to create the entity attribute visualizations (4.4-12 to 4.4-15) and all the visual graphics with explanations and comments can be found on the Kaggle platform under the query "San Francisco. Construction Sector 1980-2019.".

Datasets contain information on the issued building permits with various characteristics of the facility for which the permit is issued

Heat map visualizing attribute-columns of entities in a dataset and helps to identify relationships between pairs of variables from nearly a million records
By analyzing (with Pandas DataFrame) value data from 1,137,695 permits, it is clear that construction activity in San Francisco is closely tied to economic cycles, especially in the booming Silicon Valley technology industry. Economic booms and busts have had a significant impact on the number and value of construction projects. For example, the first peak in construction activity coincided with the electronics boom of the mid-1980s, and subsequent peaks and declines were associated with the dot-com bubble and the technology boom of recent years.

San Francisco's real estate saw cycles of sharp growth and decline across sectors, with a tech boom driving apartment demand, the dotcom bubble impacting retail space
No trends or conclusions are apparent from the table itself, provided by the San Francisco Department of Buildings. Dry numbers are not the basis for decision-making. In order to make the data visually understandable, as we discussed in the "Data Visualization" chapter, the data was visualized using various libraries from the "Loading ETL: Visualizing Results as Graphs" chapter.
In San Francisco, the majority of the $91.5 billion invested in construction and renovation, nearly 75%, is concentrated in the downtown core and within a 2 km radius of downtown, reflecting the higher density of investment in these central zones. The average cost of building permits varies significantly by neighborhood, with applications in the downtown area costing three times as much as those outside the downtown area due to higher costs of land, labor, materials, and strict building codes requiring more expensive materials for energy efficiency.

In SF, 75% of the $91.5 billion construction investment concentrated downtown, with areas like Treasure Island and Mission Bay having exceptionally high permit costs
Dataset allows to calculate average repair prices not only by house type but also by city districts and individual addresses. In San Francisco, the dynamics of home remodeling costs show distinct trends for different types of remodeling and housing. Kitchen renovations are noticeably more expensive than bathroom renovations, with the average kitchen renovation in a single-family home costing about $28,000 compared to $25,000 in a two-family home.

In SF, renovating kitchens costs nearly double that of bathrooms and homeowners needing to save $350 monthly for 15 years to cover the steadily rising costs of essential home renovations
The inflation of construction costs in San Francisco over the years can be traced by analyzing data grouped by housing type and year, showing a steady increase in the average cost of repairs since 1990 and identifying short-term 3-year cycles in the cost of repairs for multifamily buildings. The analysis highlights the variable and often unpredictable nature of construction costs in the city, which are influenced by a variety of factors including economic booms, technological advances, and specific requirements for different housing types.
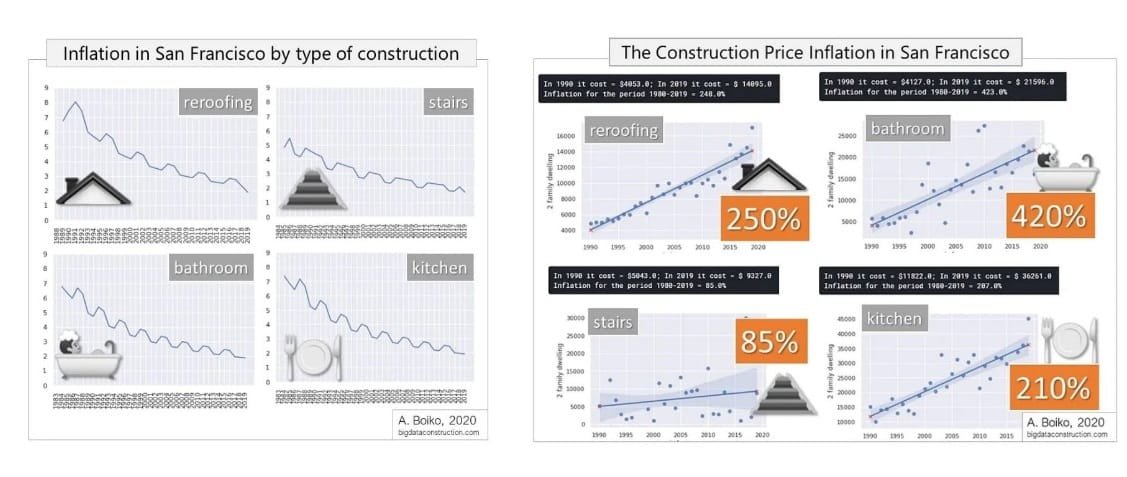
From 1980 to 2019, the cost of bathroom renovations in SF increased fivefold, while roof and kitchen renovations tripled in cost, and stair repair costs rose by only 85%
Previously, analytics creation demanded expertise in programming languages and analytical libraries; however, the advent of tools like ChatGPT has made this process accessible and engaging for everyone in the construction industry, from on-site workers to company executives.

Transitioning to visually clear and explainable building processes shifts management from manual to automated, data-driven decision-making
Just as we analysed data from the "San Francisco Building Department" tabular dataset we can visualise and analyse any data set from images and documents to data from the IOT or data from CAD (BIM) models.